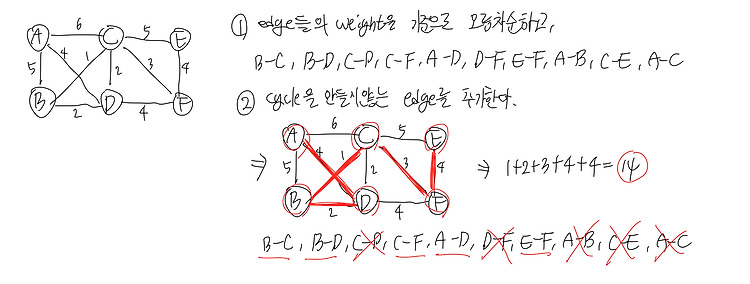
(23.03.20-22) algorithms S. Dasgupta, C. H. Papadimitriou, and U. V. Vazirani (2008) 책 읽고 정리하기 http://algorithmics.lsi.upc.edu/docs/Dasgupta-Papadimitriou-Vazirani.pdf 정리한 내용 5.1 Minimum spanning trees -> kruskal's algorithm -> cut property -> Union Find Greedy algorithm 매 순간마다의 최선의 선택을 하는 방법인데, 매순간마다의 그 최선의 선택이 결국 최종적으로 최선의 선택이 된다는 보장은 없다. top-down 방식으로 최선의 선택을 하는 것이다. 다시 말하면 아래와 같다. "solution ..
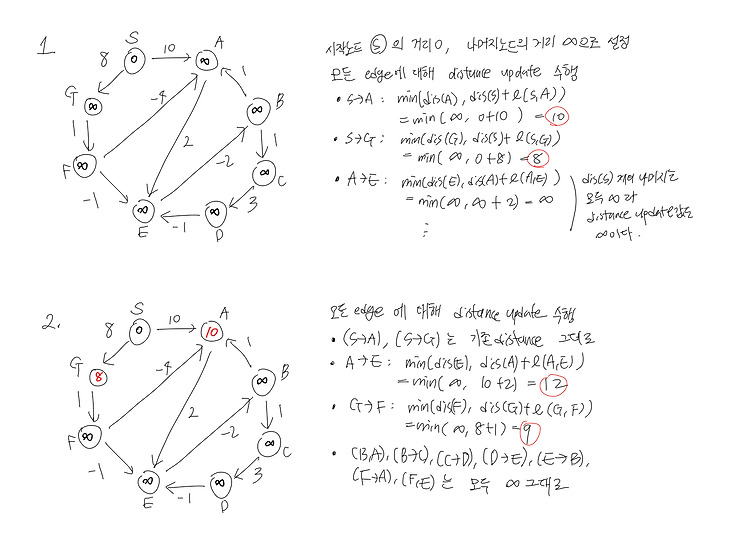
(23.03.19) algorithms S. Dasgupta, C. H. Papadimitriou, and U. V. Vazirani (2008) 책 읽고 정리하기 http://algorithmics.lsi.upc.edu/docs/Dasgupta-Papadimitriou-Vazirani.pdf 정리한 내용 4.6 Shortest paths in the presence of negative edges 4.7 Shortest paths in dags negative edges가 있는 graph에서 shortest path를 찾는 알고리즘으로는 Bellman-Ford algorithm 이 있다. - input: graph, length_e, start_node - output: distance_v - 시작 노..
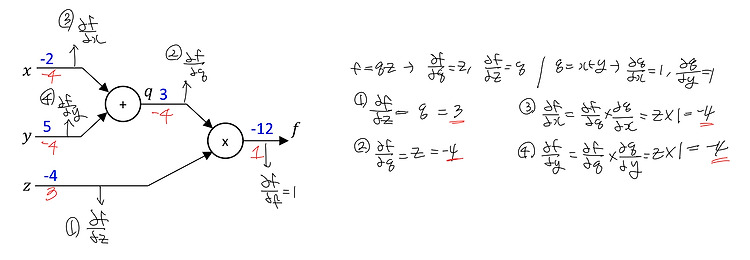
(23.03.18) 이번에는 퍼셉트론을 이용한 AND, OR, NOR, XOR gate 연산, forward/backward propagation, activation function 에 대해 정리해보았다. Perceptron - 입력 신호에 가중치가 곱해지고, 그 값들이 더해져 최종적으로 출력한다. - 활성화 함수: 출력 노드에 모인 신호들을 내보낼 때, 얼마나 강하게 내보낼지 정하는 함수로 (sigmoid, ReLU 등이 있다) - perceptron의 학습: 출력값과 목표값을 비교하여 나오는 오차를 줄이는 방향으로 가중치(w)를 조절하는 것 - hyperparameter: w의 조정 정도 (learning rate), loss function을 무엇으로 정할 것인지, ----> weight (par..
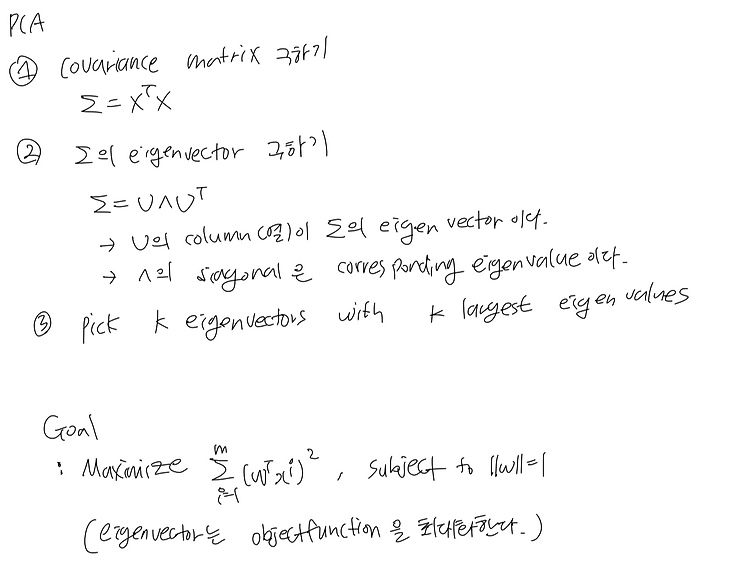
(23.03.18) 이번에 정리할 unsupervised learning 모델은 k-means clustering, PCA 이다. k-means와 pca 코드 구현한 것 > 중심점이 바뀌지 않을 때까지 2번과 3번 과정을 반복한다. - cost function: 각 데이터들과 cluster 중심점 사이 거리의 평균 - 중심점을 어디로 잡느냐에 따라 clustering 결과가 달라지는데, 학습을 여러번 돌려보거나 training set을 랜덤하게 여러번 추출하여 돌려보면 해결할 수 있다. - k를 정하는 방법으로는 elbow method가 있다 -----> k의 개수를 늘려가며 cost의 추이를 파악하고, 꺾이는 지점의 k를 사용한다. pca (principal component analysis) - pc..
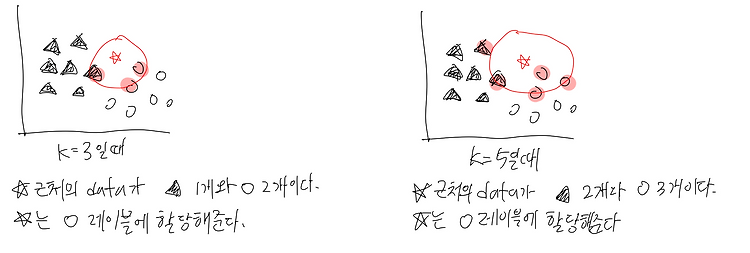
(23.03.18) 이번에 정리한 supervised learning 모델은 k-nn, SVM, decision tree, ensemble, random forest이다. SVM 관련 코드 구현 > svm의 goal: margin이 가장 큰 hyperplane 찾기 1. hard margin svm: 어떠한 data도 margin 내에 들어오지 않도록 하는 경우 margin 내의 데이터가 엄격하게 제한되기 때문에 small noise에도 민감하다. 2. soft margin svm: 일부 data는 margin 내에 들어오도록 허용하는 경우 이때는 margin내에 들어가는 data의 수를 제어하는 변수가 필요한데, 이를 slack이라고 한다. decision tree (결정트리) - classificat..
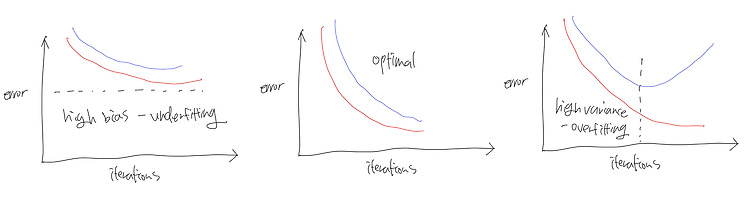
(23.03.18) overfitting (과적합) & underfitting (과소적합) overfitting problem : 모델이 training data에 지나치게 fitting하여 새로운 data에 대한 예측을 제대로 하지 못하는 것으로 일반화능력이 떨어지는 문제이다. - 학습하는 과정에서 error를 무조건 낮게 학습시키는 것은 overfitting을 초래할 수 있다. - overfitting 해결책 #1. feature 수 줄이기 - 어떤 feature를 유지할 것인가? -> model selection algorithm - overfitting 해결책 #2. regularization: feature들을 모두 유지하는 방법 - parameter(weights)들을 모두 조절해서 고차원 f..
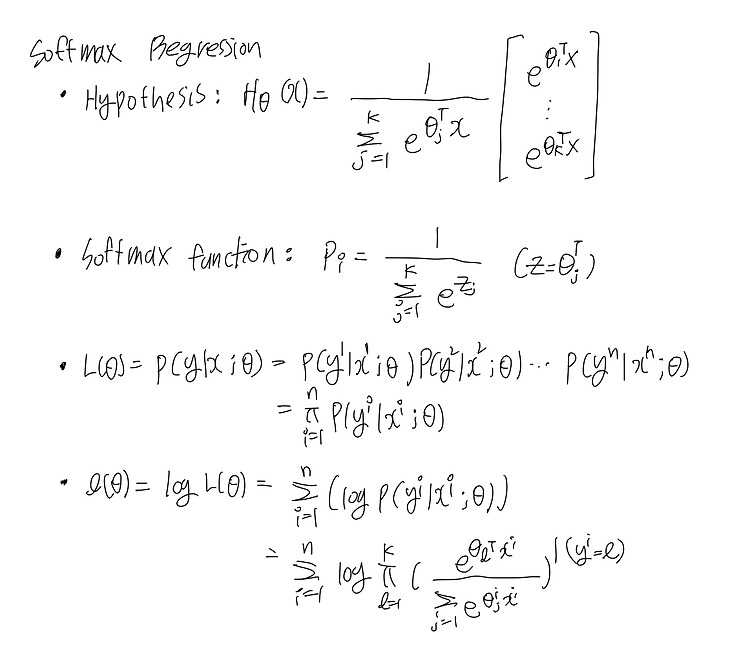
(23.03.18) 추후 더 자세히...... 작성할 예정 softmax regression - softmax regression은 multi-class classification을 수행한다. - logistic regression classifier를 각 class i에 대해서 학습시키고, y=i일 확률을 예측한다. 새로운 input x에 대해 h(x)를 최대화하는 i를 찾는다. 그러면, 이는 input x는 해당 class에 속한다고 예측하는 것이다.
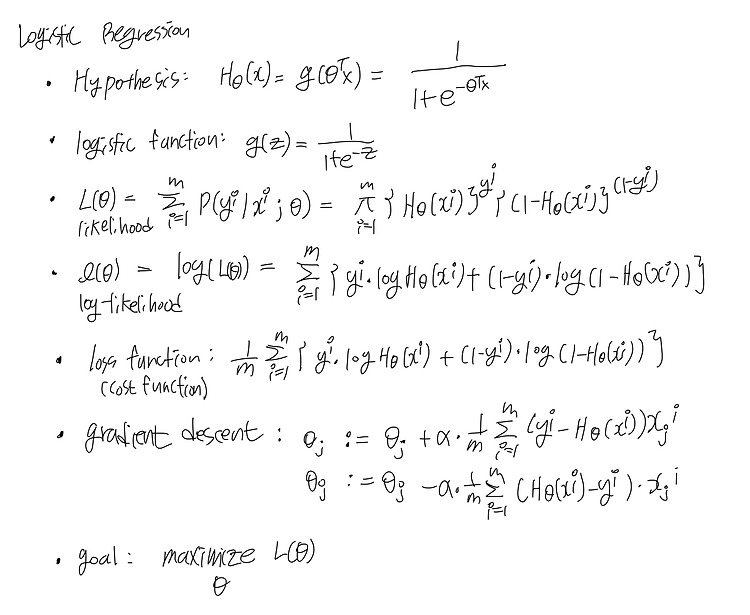
(23.03.18) binary classification -> logistic regression multi-class classification -> softmax regression logistic regression 관련 코드 구현한 것 >
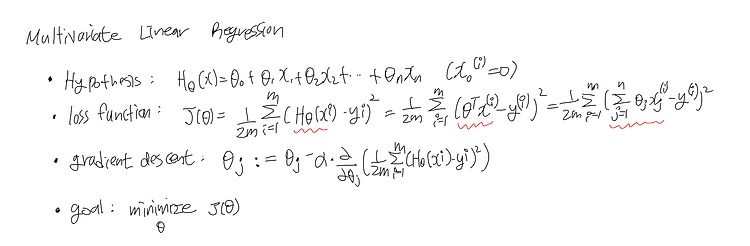
(23.03.17) Multivariate Linear Regression : 다변량 선형 회귀 - linear regression에서는 feature가 하나였다면, multivariate linear regression에서는 feature가 여러 개이다. 가설함수, 손실함수, 경사하강법에 관련된 식들을 정리하면 아래와 같다. Locally-weighted linear regression - linear model을 여러 개 사용해서 non-linear predeictions 처럼 만들어주는 방법이다. - loss funciton은 아래와 같다. polynomial regression : 다항 회귀 - 다항 회귀는 feature는 하나인데, 가설함수 linear하지 않다. feature인 x의 지수가 다..
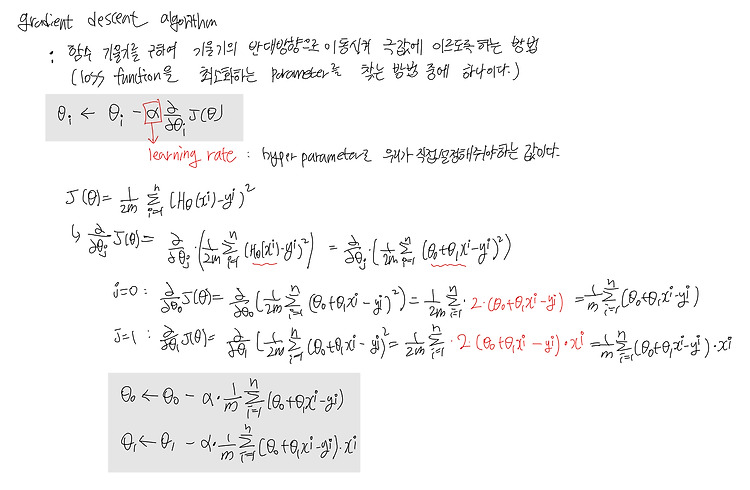
(23.03.17) suervised learning, 기계학습의 대표적인 방법인 linear regression 을 정리해보았다. linear regression 관련된 코드 구현 > hypothesis 가설 함수를 세우고 (parameter) , loss function을 세운다. 기본적인 가설함수로는 parameter가 2개인 일차함수 형태가 있다. loss function은 주로, 예측값과 실제값 차이의 제곱을 계산하는 함수로 둔다. (mean square loss) > loss function의 기울기를 계산하여 loss function을 최소화하는 지점을 찾고자 gradient descent 기법을 사용한다. > loss function을 최소화하는 parameter를 찾아 데이터를 가장 잘 ..
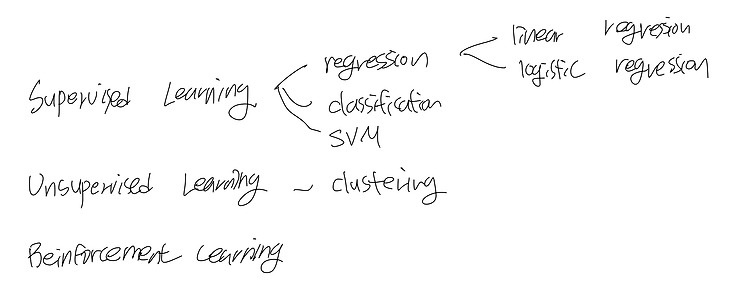
(23.03.17) 머신러닝 내용 정리하기 기계학습, 데분통 수강하며 정리했던 내용들 업로드 하는 중~~ 3월 안에 다 정리하는 것을 목표로 함!! Machine Learning - 전통적인 프로그래밍과는 다르게 컴퓨터에게 하나하나 지시하는 것이 아니라, 어떤 데이터가 주어졌을 때 그 속에서 규칙을 찾아내어 문제를 해결할 수 있도록 하는 것이다. - 그림으로 표현한다면 아래와 같다. Machine learning은 아래의 그림과 같이 분류해볼 수 있다. 그러나 현재 classification의 경우, supervised learning로 해결할 수도 있지만, unsupervised learning으로도 해결하고자하는 연구가 많이 나오고 있어 명확하게 task를 나누기엔 애매하다.
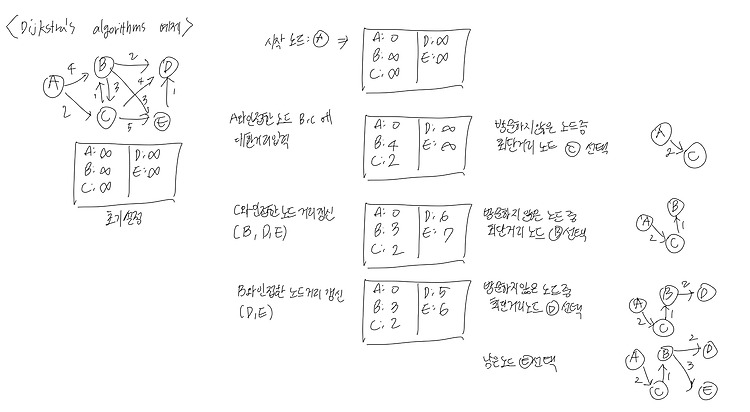
(23.03.17) algorithms S. Dasgupta, C. H. Papadimitriou, and U. V. Vazirani (2008) 책 읽고 정리하기 http://algorithmics.lsi.upc.edu/docs/Dasgupta-Papadimitriou-Vazirani.pdf 정리한 내용 4.4 Dijkstra's algorithm 4.5 Prority queue implementations Dijkstra's algorithm은 BFS를 좀더 일반적인 graph로 가져온 것인데, BFS와는 다르게 edge의 length가 positive integers로 구성되어있다. Dijkstra's algorithm 수행 예제 priority queue - 우선순위 큐 heap - heap di..

(23.03.16) (최종 업데이트: 23.05.14) https://github.com/gompaang/algorithm_python GitHub - gompaang/algorithm_python Contribute to gompaang/algorithm_python development by creating an account on GitHub. github.com DFS, BFS는 탐색 알고리즘의 대표적인 2가지 알고리즘이다. DFS,BFS 구현시 알아두어야하는 자료구조 #reference: https://data-marketing-bk.tistory.com/44 #depth-first search #1. dfs by python list (stack) def dfs_list(graph, start..
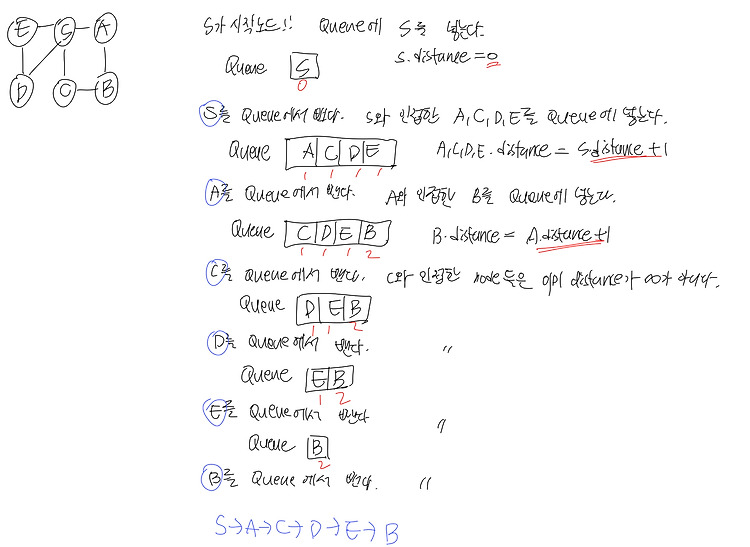
(23.03.16) algorithms S. Dasgupta, C. H. Papadimitriou, and U. V. Vazirani (2008) 책 읽고 정리하기 http://algorithmics.lsi.upc.edu/docs/Dasgupta-Papadimitriou-Vazirani.pdf 정리한 내용 4.1 Distances 4.2 Breadth-first search 4.3 lenghts on edges BFS 예제 수업 들으면서 정리해두었던, DFS, BFS 개념과 예제 https://hey-stranger.tistory.com/153 [알고리즘] Network flow : BFS & DFS (2021.11.12) 알고리즘 수업들으면서 정리하기 19탄 w10-3 녹화강의, w11-1 실강 BFS..
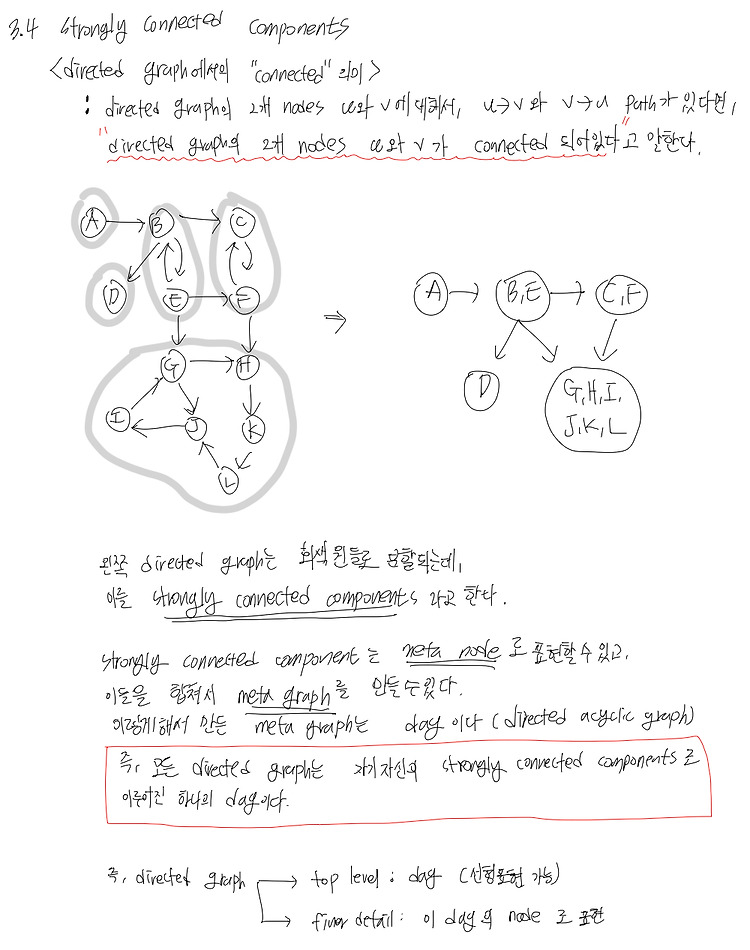
(23.03.15) algorithms S. Dasgupta, C. H. Papadimitriou, and U. V. Vazirani (2008) 책 읽고 정리하기 http://algorithmics.lsi.upc.edu/docs/Dasgupta-Papadimitriou-Vazirani.pdf 정리한 내용 3.4 Strongly connected components